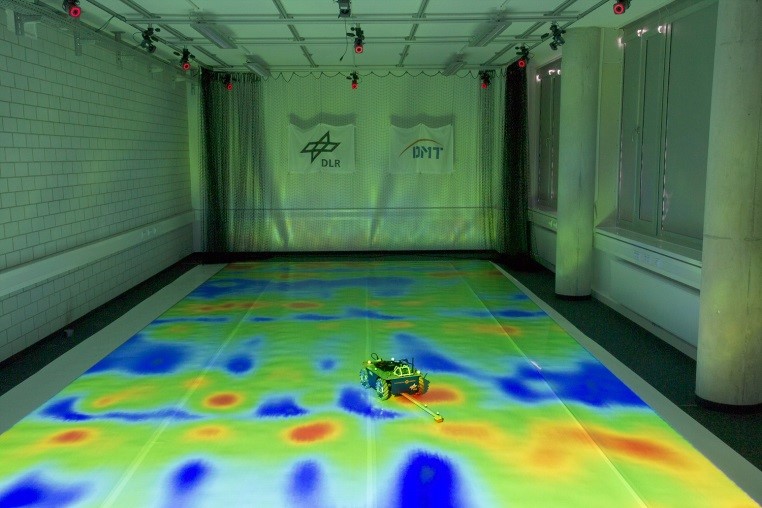
ULearn4Mobility – Ubiquitous Spatio-Temporal Learning for Future Mobility
- Contact:
- Funding:
- Partner:
- Startdate:
October 2020
- Enddate:
September 2022
The project considers the development of an algorithmic framework for learning
large-scale spatio-temporal systems related to future mobility. With the deployment of 5G
mobile networks, ubiquitous sensor fusion for mapping physical phenomena becomes
technologically feasible. Improved computational power is available on crowdsensing
agents such as cell phones, cars, and trains. Real-world physical phenomena often
involve variables that vary in both space and time. The magnetic field, for instance, can be
altered drastically by moving ferromagnetic objects. Modeling such spatio-temporal
physical phenomena is nontrivial due to the underlying complex dynamics. Therefore, we
develop learning approaches based on Gaussian processes (GPs) that facilitate efficient
spatio-temporal learning in distributed architectures. The GPs with a distributed
architecture allow modeling physical phenomena on site at the individual nodes and
instant inference. The key application of the project is learning the time-varying magnetic
field to localize agents via multi-sensor fusion. A GP-based reconstruction approach
requires spatial coordinates of the training and test data sets. Therefore, we tightly couple
the location estimation with the spatio-temporal model in a joint learning approach, based
on which a simultaneous positioning and map learning (SPAML) algorithm will be
developed. Mobile localization based thereon relies on crowdsensing and is independent
of global navigation satellite systems (GNSS). Thus, it is expected to work robustly in
GNSS-denied scenarios. The proposed spatio-temporal learning scheme is self-contained
and of generic utility for future mobility applications and related engineering problems.
large-scale spatio-temporal systems related to future mobility. With the deployment of 5G
mobile networks, ubiquitous sensor fusion for mapping physical phenomena becomes
technologically feasible. Improved computational power is available on crowdsensing
agents such as cell phones, cars, and trains. Real-world physical phenomena often
involve variables that vary in both space and time. The magnetic field, for instance, can be
altered drastically by moving ferromagnetic objects. Modeling such spatio-temporal
physical phenomena is nontrivial due to the underlying complex dynamics. Therefore, we
develop learning approaches based on Gaussian processes (GPs) that facilitate efficient
spatio-temporal learning in distributed architectures. The GPs with a distributed
architecture allow modeling physical phenomena on site at the individual nodes and
instant inference. The key application of the project is learning the time-varying magnetic
field to localize agents via multi-sensor fusion. A GP-based reconstruction approach
requires spatial coordinates of the training and test data sets. Therefore, we tightly couple
the location estimation with the spatio-temporal model in a joint learning approach, based
on which a simultaneous positioning and map learning (SPAML) algorithm will be
developed. Mobile localization based thereon relies on crowdsensing and is independent
of global navigation satellite systems (GNSS). Thus, it is expected to work robustly in
GNSS-denied scenarios. The proposed spatio-temporal learning scheme is self-contained
and of generic utility for future mobility applications and related engineering problems.